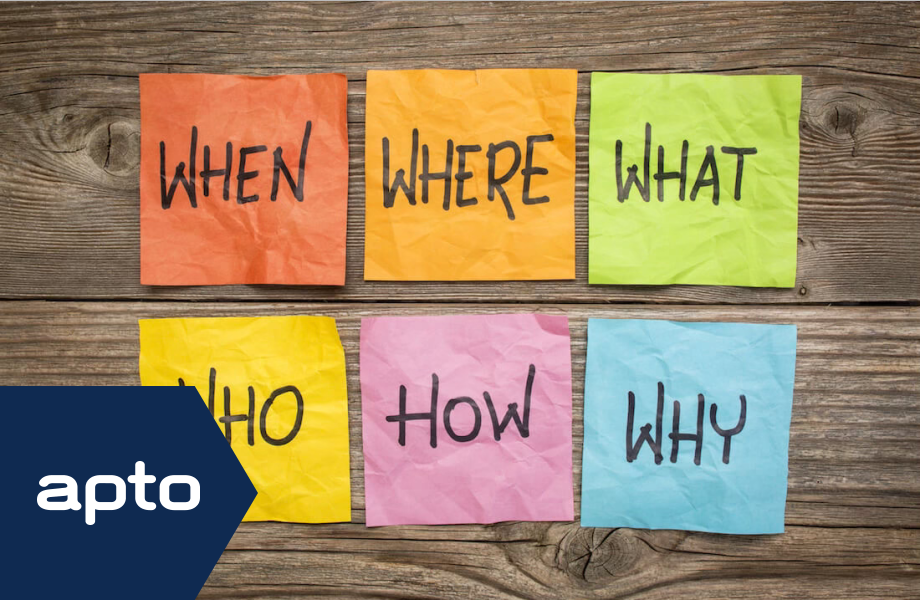
Understanding your data is a fascinating and often complex challenge when explored in depth. However, from a high-level perspective, you can gain valuable insights by breaking it down into five standard components: where, how, why, who, and what.
Where?
Let’s start with where the data is generated. This is a crucial aspect both from a governance standpoint and in understanding the data’s available metrics and overall significance. Knowing the geographical origin of your data is especially important for meeting compliance requirements and can influence what you’re able to do with it.
It also sheds light on the kind of metrics you can derive. If the data is generated internally, it’s important to explore how users are creating it, what volumes are involved and how that shapes its meaning. If you’re receiving data from external sources, understanding how it is collected adds context and purpose to the metrics. It’s not just about what the metric is; it’s about giving that metric proper context, scope and meaning.
How?
Next is how the data is generated. This often gets overlooked, especially when attention is focused on data ingestion or transformation. Yet understanding how data is created is a vital part of gaining deep insight.
Consider the sources generating the data and the output types they use. This overlaps with the where, adding another layer of context. Exploring how data is generated gives you a richer understanding without getting bogged down in technicalities.
Then there’s the next layer: why it’s useful and important. We often see data strategies that focus on ingesting everything, with little thought given to the actual value or significance of that data.
When you view data through the lens of where it’s generated, you gain human context. Thinking about how it’s generated provides technical context, insight into systems and tooling.
Why + Who?
Now ask: why is this data useful? Is it important? Does it offer functional value, or would treating it as an operational metric be excessive? These questions shape how you manage and prioritise data and whether it warrants attention through platforms like Splunk.
From there, consider who is using it. If it’s already in use, break that down by stakeholder. If not, consider who should be using it. What are the ideal use cases?
What?
Finally, we reach the last component: what the data can do. Your data can always do more than its initial value suggests. By thinking about inference, dependance, and secondary characteristics we can unbound data. What data can do is open ended rather than a closed answer, you should always be considering you data and its applications as a living concept!
Conclusion
We’ve recently worked with several clients using car park data. At first glance, you might assume it’s mainly relevant to staff monitoring the car parks. But by applying these questions – where it’s generated (in the car park, within the business), how it’s generated (via the movement of cars and passengers), why + what it’s useful (it reflects the volume of people arriving and has financial relevance) you start to see a bigger picture.
Understanding it in this way reveals that not just car park attendants, but also customer service teams and capacity planners might benefit from the data. This example clearly paints why understanding your data and its components is so important, and how our high level thinking process can help you consider the true value your data can bring.
-
26 June 2025
SIEM Platform Management
-
26 June 2025
Using Your Data Effectively in Enterprise Security
-
26 June 2025
Data Discovery Process
See how we can build your digital capability,
call us on +44(0)845 226 3351 or send us an email…